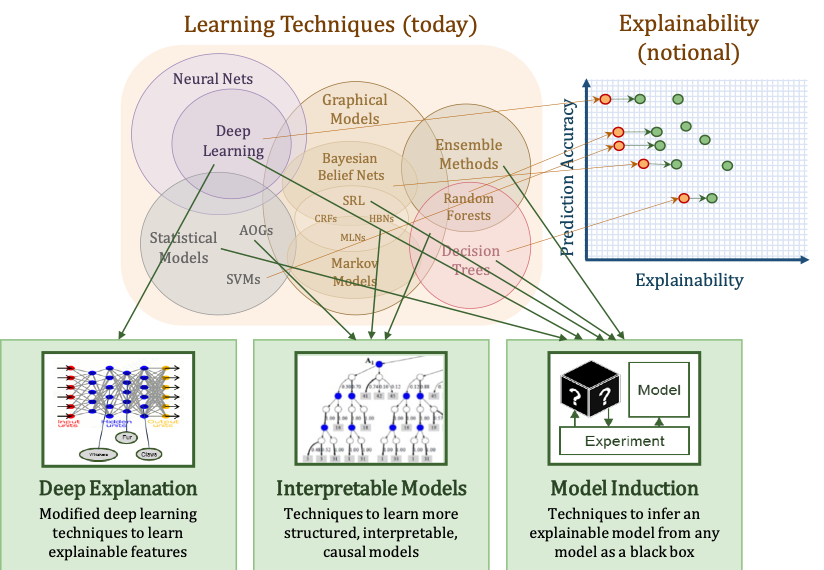
Source: “Explainable Artificial Intelligence (XAI)"
As the breakthrough of Deep Neural Nets (DNNs) accompanied by the advanced computing power in recent years, artificial neural network models are again back to the stage and extensively applied in industries as well as widely studied in the academics. DNNs are known for the (linear/nonlinear) complex transformation throughout many hidden layers. Therefore, given a well-trained DNN that makes good classifications, it is still a mystery the inference process within the model, making DNNs also known as black boxes. Without any causal inferences from the output, i.e., the classified results, to the input factors, the creditability of a DNN model is insufficient and the potential for the model to be implemented reduces. As shown in the figure above, DARPA started the fundamental research in studying the modeling explainability according to the DNN model properties. In many application domains, the factors used in the input layer are meaningful and can be self-explained intuitively. It is extremely critical to trace back to the input layer for the identification of key contributors. Especially, in those industries where the model causality is regarded as important as the predictability, such as the yield analysis and the process control in the manufacturing context, Explainable AI (XAI) would be the key to open massive implementation of DNN models.