Student:Kuo, Tzu-Hsuan
Thesis Advisor:Professor Wu, Cheng-Hung
Thesis Topic :Demand Parameters Estimation and Dynamic Pricing Considering Reference Prices
Abstract:
This research applies deep learning method in demand parameters estimation and dynamic pricing to train a model that can make accurate estimation in unknown market environments and conduct decision optimization in real time.
Good dynamic pricing strategy considering reference prices are crucial to sellers with short selling season. Mean of customers’ valuation are usually difficult to quantify and estimate. Being unable to estimate demand parameters efficiently will lead to bad pricing strategy and considerable profit loss. Our study proposes a model to solve the difficulties mentioned above.
We consider a dynamic pricing problem in a market selling single perishable product with the goal of maximizing expected profits. We take mean of customers’ valuation and reference prices into the consideration of dynamic pricing with the application of multinomial logit model, solving the optimal pricing strategies under all possible states. Then we use a large amount of historical sales data to develop a long short-term memory neural network which can precisely estimate mean of customers’ valuation in unknown market environments. Finally, we apply adaptive training to improve the performance of LSTM’s estimation and pricing strategy, let model be self-adaptive to current market environments by utilizing real-time sales data, then use discrete events simulation to verify our model’s accuracy and generalization under unknown environments. The result indicates that our model performs well in a variety of unknown market environments.
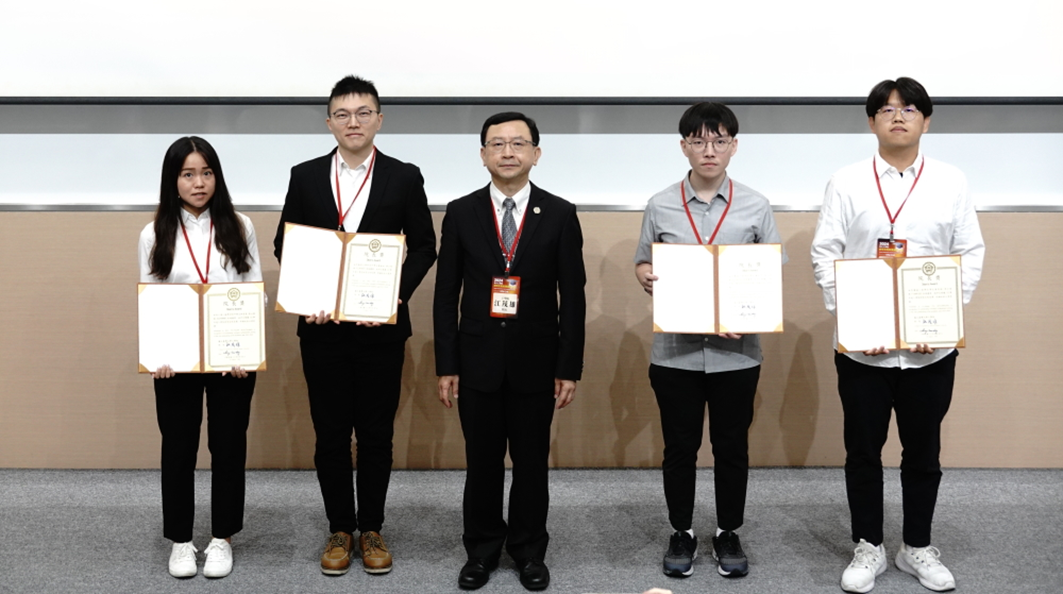
Poster:
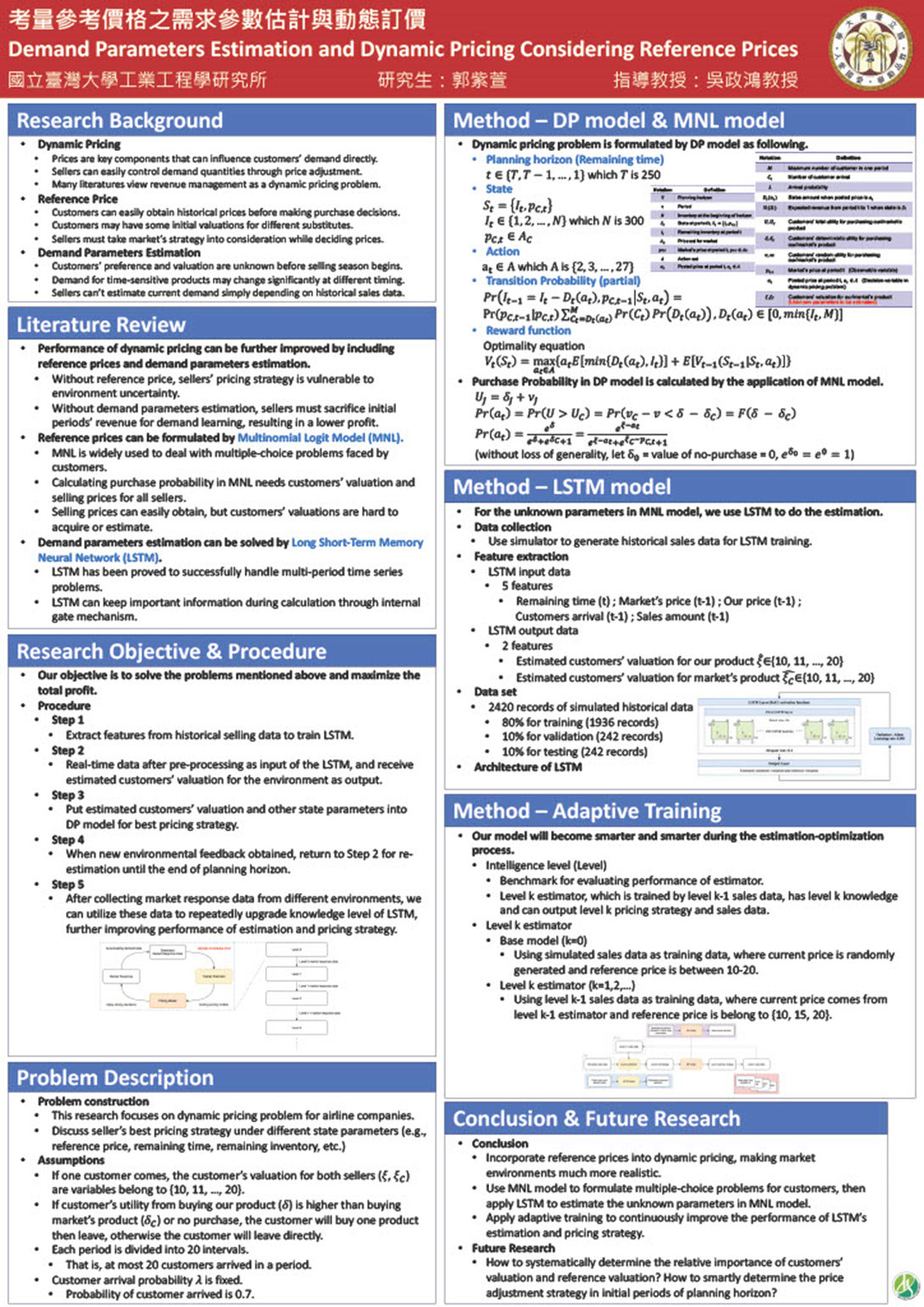